More about the competition
Objective of the competition:
The Lacuna Fund has launched a competition aimed at developing an AI model capable of detecting and classifying malaria parasites in blood samples. Their goal is to enable early and accurate malaria diagnosis, ensuring timely treatment, saving lives, and helping healthcare workers to focus on patient care and treatment planning.
Reasons for participation:
One of our missions is to advance AI for sustainability and solve humanitarian issues. Participation in this competition aligns with our goals of developing technology for impactful solutions, in this case in the context of health and helping underserved communities.
Dataset Composition and Source
Data Collection Process:
The dataset includes thousands of blood sample images gathered from hospitals in Uganda and Ghana. These samples are annotated, providing a foundation for training AI models to accurately detect and classify malaria parasites. It features both thick and thin blood smears, commonly used techniques in malaria diagnostics. The images were captured using smartphones mounted on microscopes, offering a practical and cost-effective approach.
Data Source:
The Lacuna Fund collaborated with hospitals in Uganda and Ghana to develop this dataset, ensuring broad representation of the populations most affected by malaria. All data was collected anonymously, ensuring ethical practices and privacy of the patients.
Ethics, Privacy, and Bias Mitigation
The dataset follows strict ethical guidelines:
Anonymity: Blood samples are anonymized to protect patient identities.
Bias: Ensuring data represents the affected populations to avoid model bias.
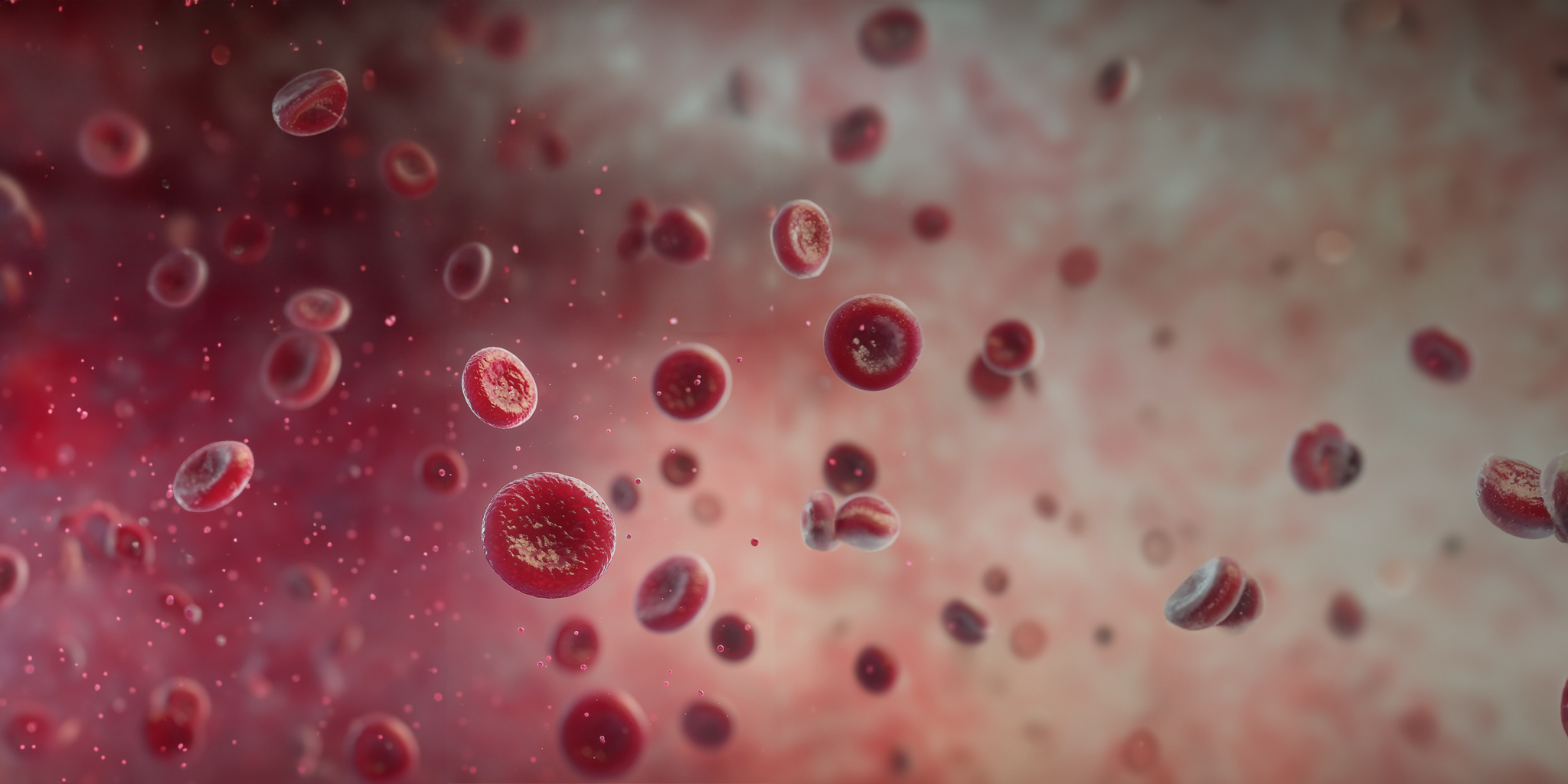
The Lacuna Fund
The Lacuna Fund mobilizes resources to develop high-quality, locally sourced, and ethically collected datasets that address challenges in low- and middle-income countries.Lacuna Fund enables the creation of new datasets for underrepresented populations, enhances existing datasets for broader applicability, and updates outdated datasets. These datasets are locally developed, ethically sourced, and openly accessible, promoting data equity on an international scale.
Lacuna Fund provides the necessary resources to either create new datasets that address underserved populations or critical issues, enhance existing datasets to ensure greater representation, or update outdated datasets, to stay fresh and reliable.
Model Implementations
The proposed malaria detection model can be implemented using a simple setup: a microscope, a smartphone, and a custom app.
Prepare the Blood Sample: Place the blood slide under the microscope.
Attach the Phone: Position the smartphone camera on top of the microscope.
Open the App: Launch the malaria detection app.
Capture the Image: Take a picture of the blood sample using the app's interface.
Upload and Analyze: The app processes the image using the AI model to identify malaria parasites.
Detection Output:
Parasite Identification: Boxes appear around detected parasites with labels indicating the parasite stage (e.g., trophozoite).
Confidence Scores: Probability values accompany detections to indicate result reliability.