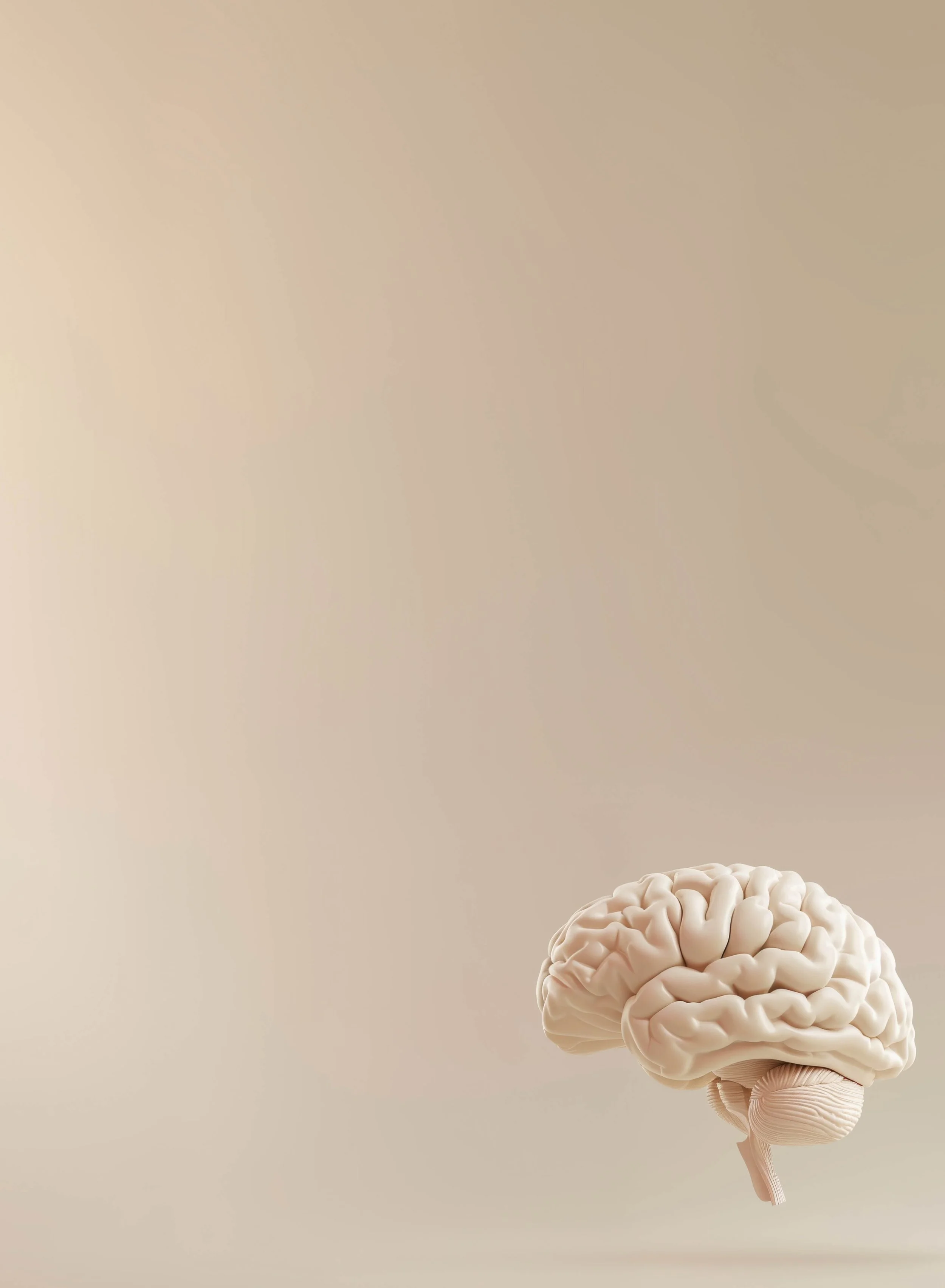
Classifying Harmful Brain Activity
Classifying seizures and other harmful brain patterns by using electrical brain activity data
About the competition
What happens inside the mind of someone in a coma, or someone who suffers from a seizure? This is what neurologists analyze using electroencephalography (EEG). It is commonly used to diagnose various neurological disorders such as epilepsy and helps to identify abnormalities in brain waves. This technology serves as a window into the brain’s inner workings. When the neurons in your brain communicate, they create small electrical signals that show up in a similar way as an audio recording. When someone suffers from a seizure, a big electrical storm occurs. These patterns are unusual and detectable through EEG. In this competition, we will develop an AI that can help a neurologist with analyzing the EEG data.
Harvard Medical School
The competition is hosted by Harvard Medical School, the graduate medical school of Harvard University. They are joined by the Sunstella Foundation, Persyst, Jazz Pharmaceuticals, and the Clinical Data Animation Center (CDAC), whose research aims to help people preserve and enhance brain health.
Relevance
Neurologist and expert in EEG, Robert van den Berg, taught us the importance of using AI to help analyze EEG data. He affirmed that using this will support decision-making. Additionally, it can serve as an early warning system when a patient is being monitored long-term. Essentially, it will reduce workload and result in better care. He confirmed that research has been done on using AI for EEG analyses. However, the use of AI in health care still lacks trust which slows down the process of implementation. Many patients worry that algorithms can’t meet their medical needs well enough. To change this, we need AI’s that show expert-level accuracy or even outperform experts. By providing accuracy and reliability our models can gain trust for use in settings like healthcare.
“ By providing accuracy and reliability our models can gain trust for use in settings like healthcare.
Technical details
In the data provided for this competition, Medical expert annotators reviewed 50-second-long EEG samples combined with extracted frequency spectrograms. They focused on spotting harmful brain activity within each sample. Interestingly, even these experts found it tough to agree on the right labels when examining the data. For example, it is a common occurrence that half of the experts vote for a Seizure, and the other half vote for Other harmful behaviour. Therefore, our models should match the expert’s uncertainty and label the data identically. That will result in the highest score. The team researches and develops state-of-the-art recurrent neural networks which can pick up on the rhythmic patterns of harmful events in EEG data.
UN Sustainable Development Goals
This competition contributes to the UN Sustainable Development Goal #3: Good Health and Well-being. Our AI model will help neurologists in treating their patients faster and more accurately. It will also help justify the research and development in using AI in the healthcare field.
Status: Finished
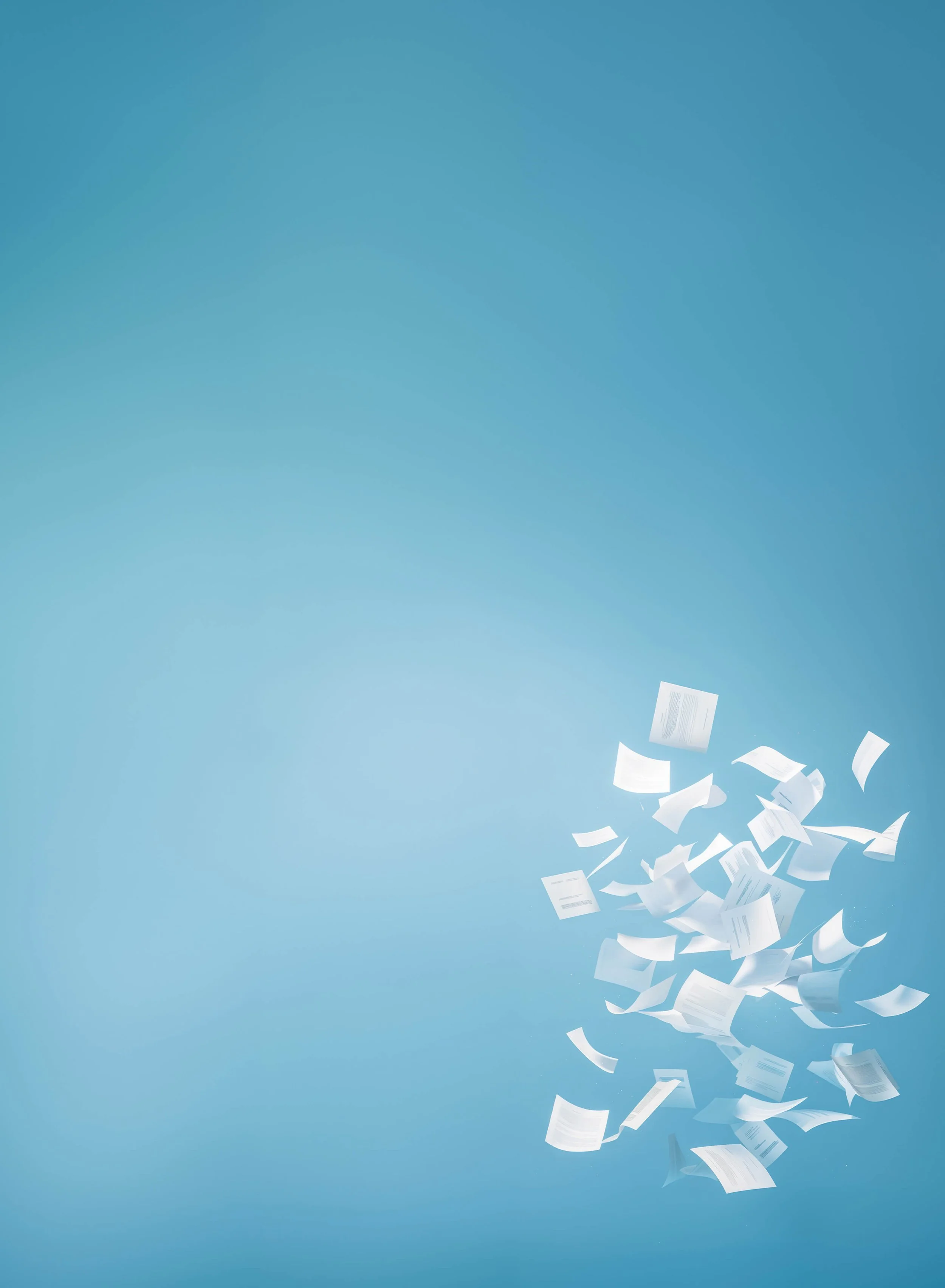
Open government act
Optimising the Woo process by providing a clear overview of the requested documents
About the project
During this project, led by PhD candidate Max Muller and organised by I-Partnership of the Dutch Government and the National Archive, Team Epoch develops an AI that will organise archived documents in a coherent time framework. This will disclose information by the Dutch government more efficiently and contribute to the Woo law of the government.
National Archive
The National Archive supports the Dutch government by permanently retaining information. Its mission revolves around ensuring universal access to information. By prioritizing the principles of findability, accessibility, readability, and interpretability, the National Archive aims to fulfil its mandate of facilitating public access to government documents.
Relevance
In May 2022, the Dutch Government introduced a new law: the ‘Wet Open Overheid’. This law aligns with Article 19 of the Universal Human Rights: the right to information.
However, the existing archival system faces challenges due to the lack of a standardized organizational structure. Currently, documents that arrive at the National Archive are not structured in a general contextual system, resulting in an accumulation of unstructured documents where the links between documents remain unclear. Consequently, there is a huge delay in processing information requests. The legal delivery time of information upon request is 4 weeks, however this often exceeds up to months. These requests are often made by investigative data journalists whose goal it is to inform the public.
The poor structure of the documents leads to contextual unclarity. To understand information held by the documents, a structure of timelines is required, to interpret the information. These timelines will help us understand the context the document was produced in, think of time, place and the people involved. Furthermore, it will show how separate documents are interlinked.
The goal of this project is to convince the government of the usability of the AI tool by producing a working example. Team Epoch will show that it is possible to write an AI that can structure the documents in timelines and will thereby act as a catalyst for further development.
“ Epoch’s AI will act as a catalyst in optimising the Woo requests.
Technical details
Our dataset consists of thousands of WOB-request dossiers containing millions of documents. Our task is to create an AI that, given a dossier of documents, can organize these in a narrative map.
A narrative map is a structure to organize events from a story in a visually rich way. It’s a graph where each event is its own node and the paths between the nodes represent similarity. The main advantage of using a narrative map over a simple timeline is that there can be multiple paths within a story that could follow certain people, locations, or subtopics. For example, a narrative map about the COVID-19 pandemic could start at the first encounter of the virus in Wuhan, China and branch into different subtopics from there. One branch would describe how the virus spread over the world, another might dive into how China handled the situation, another branch could explore the development of vaccines, etc.
Unlike our previous competitions, this project does not come with training labels. This means that the progress made by the team cannot be tested on accuracy with a test data set. The narrative maps are also difficult to judge, which makes it hard to say whether a map “makes sense” or if one map is better than the other. In addition, there is no consensus on what makes a map “good”, which makes it a lot more difficult to train a model.
UN Sustainable Development Goals
This project directly contributes to SDG 16: Peace, Justice, and Strong Institutions. By improving the accessibility and transparency of governmental information, Team Epoch's project aligns with the overarching goal of gaining public trust and engagement in governance processes, thus making the government a strong institution.